《計(jì)量經(jīng)濟(jì)學(xué)》ch-03-wooldridg
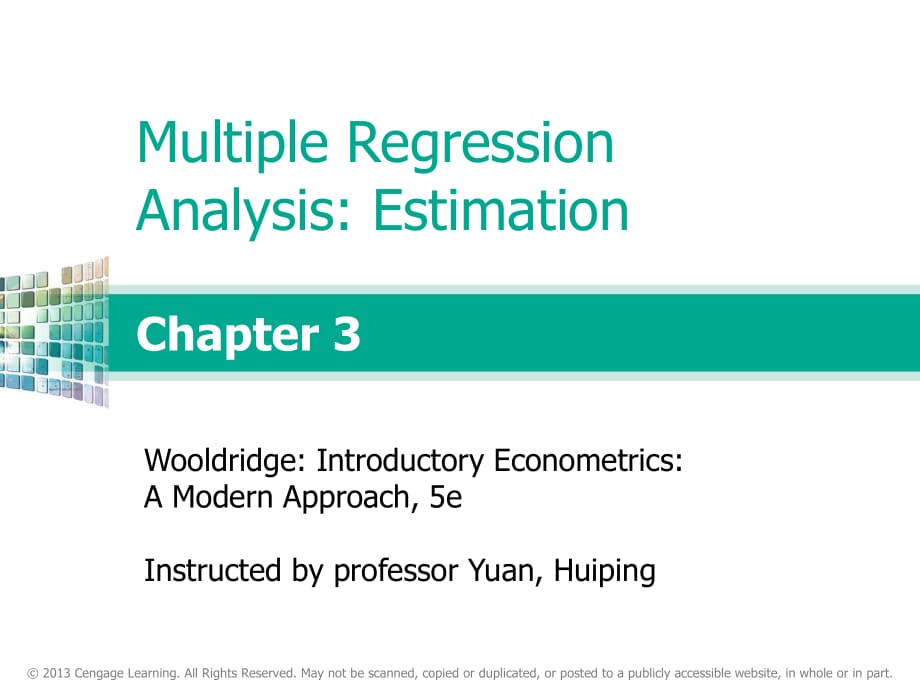


《《計(jì)量經(jīng)濟(jì)學(xué)》ch-03-wooldridg》由會(huì)員分享,可在線閱讀,更多相關(guān)《《計(jì)量經(jīng)濟(jì)學(xué)》ch-03-wooldridg(53頁(yè)珍藏版)》請(qǐng)?jiān)谘b配圖網(wǎng)上搜索。
1、 2013 Cengage Learning. All Rights Reserved. May not be scanned, copied or duplicated, or posted to a publicly accessible website, in whole or in part. Chapter 3 Multiple RegressionAnalysis: EstimationWooldridge: Introductory Econometrics: A Modern Approach, 5eInstructed by professor Yuan, Huiping 2
2、013 Cengage Learning. All Rights Reserved. May not be scanned, copied or duplicated, or posted to a publicly accessible website, in whole or in part. CHAPTER 3 Multiple RegressionAnalysis: Estimation3.2 Mechanics and Interpretation of OLS3.3 The Expected Value of the OLS Estimators3.4 The Variance o
3、f the OLS Estimators3.5 Efficiency of OLS: The Gauss-Markov Theorem3.1 Motivation for Multiple Regression3.6 Some Comments on the Language of Multiple Regression AnalysisAssignments: Promblems 7, 9, 10, 11, 13, Computer Exercises C1, C3, C5, C6, C8 The End 2013 Cengage Learning. All Rights Reserved.
4、 May not be scanned, copied or duplicated, or posted to a publicly accessible website, in whole or in part. Definition of the multiple linear regression modelDependent variable,explained variable, response variable, Independent variables,explanatory variables,regressors, Error term,disturbance,unobs
5、ervables,Intercept Slope parametersExplains variable in terms of variables “ 3.1 Motivation for Multiple Regression (1/5)CHAPTER 3 Multiple RegressionAnalysis: Estimation Chapter End 2013 Cengage Learning. All Rights Reserved. May not be scanned, copied or duplicated, or posted to a publicly accessi
6、ble website, in whole or in part. Motivation for multiple regressionIncorporate more explanatory factors into the modelExplicitly hold fixed other factors that otherwise would be in Allow for more flexible functional formsExample: Wage equation Hourly wage Years of education Labor market experienceA
7、ll other factorsNow measures effect of education explicitly holding experience fixed CHAPTER 3 Multiple RegressionAnalysis: Estimation3.1 Motivation for Multiple Regression (2/5) Chapter End 2013 Cengage Learning. All Rights Reserved. May not be scanned, copied or duplicated, or posted to a publicly
8、 accessible website, in whole or in part. Example: Average test scores and per student spendingPer student spending is likely to be correlated with average family income at a given high school because of school financingOmitting average family income in regression would lead to biased estimate of th
9、e effect of spending on average test scoresIn a simple regression model, effect of per student spending would partly include the effect of family income on test scoresAverage standardizedtest score of school Other factorsPer student spendingat this school Average family incomeof students at this sch
10、oolCHAPTER 3 Multiple RegressionAnalysis: Estimation3.1 Motivation for Multiple Regression (3/5) Chapter End 2013 Cengage Learning. All Rights Reserved. May not be scanned, copied or duplicated, or posted to a publicly accessible website, in whole or in part. Example: Family income and family consum
11、ptionModel has two explanatory variables: inome and income squaredConsumption is explained as a quadratic function of incomeOne has to be very careful when interpreting the coefficients:Family consumption Other factorsFamily income Family income squaredBy how much does consumptionincrease if income
12、is increased by one unit? Depends on how much income is already there CHAPTER 3 Multiple RegressionAnalysis: Estimation3.1 Motivation for Multiple Regression (4/5) Chapter End 2013 Cengage Learning. All Rights Reserved. May not be scanned, copied or duplicated, or posted to a publicly accessible web
13、site, in whole or in part. Example: CEO salary, sales and CEO tenureModel assumes a constant elasticity relationship between CEO salary and the sales of his or her firmModel assumes a quadratic relationship between CEO salary and his or her tenure with the firmMeaning of linear“ regressionThe model
14、has to be linear in the parameters (not in the variables)Log of CEO salary Log sales Quadratic function of CEO tenure with firmCHAPTER 3 Multiple RegressionAnalysis: Estimation3.1 Motivation for Multiple Regression (5/5) Chapter End 2013 Cengage Learning. All Rights Reserved. May not be scanned, cop
15、ied or duplicated, or posted to a publicly accessible website, in whole or in part. CHAPTER 3 Multiple RegressionAnalysis: Estimation3.2 Mechanics and Interpretation of OLS3.2.2 Interpreting the OLS Regression Equation3.2.3 OLS Fitted Values and Residuals3.2.1 Obtaining the OLS Estimates3.2.4 A “Par
16、tialling Out” Interpretation of Multiple Regression3.2.5 Comparison of Simple and Multiple Regression Estimates3.2.6 Goodness of Fit3.2.7 Regression through the Origin Chapter End 2013 Cengage Learning. All Rights Reserved. May not be scanned, copied or duplicated, or posted to a publicly accessible
17、 website, in whole or in part. OLS Estimation of the multiple regression modelRandom sampleRegression residualsMinimize sum of squared residuals Minimization will be carried out by computer CHAPTER 3 Multiple RegressionAnalysis: Estimation3.2.1 Obtaining the OLS Estimates (1/2) S ctionChapt r End 20
18、13 Cengage Learning. All Rights Reserved. May not be scanned, copied or duplicated, or posted to a publicly accessible website, in whole or in part. CHAPTER 3 Multiple RegressionAnalysis: Estimation 0 1 22 0 1 1 , ,., 0 1 11 0 1 10 1 1 min .first order conditions (normal equations) . 0 . 0 . 0popula
19、tionk i i i k iki i k iki i i k ikik i i k iku y x xy x xx y x xx y x x 11sample moments: moments: 0 0 0 for 1,.,0 for 1,., iij ijE u n un x u j kE x u j k 3.2.1 Obtaining the OLS Estimates (2/2) S ctionChapt r End 2013 Cengage Learning. All Rights Reserved. May not be scanned, copied or duplicated,
20、 or posted to a publicly accessible website, in whole or in part. Interpretation of the multiple regression modelThe multiple linear regression model manages to hold the values of other explanatory variables fixed even if, in reality, they are correlated with the explanatory variable under considera
21、tionCeteris paribus“-interpretationIt has still to be assumed that unobserved factors do not change if the explanatory variables are changedBy how much does the dependent variable change if the j-thindependent variable is increased by one unit, holding all other independent variables and the error t
22、erm constantCHAPTER 3 Multiple RegressionAnalysis: Estimation3.2.2 Interpreting the OLS Regression Equation (1/3)1 1 2 22 1 1Let 0. .If . 0 and 1, then .k kku y x x xx x x y S ctionChapt r End 2013 Cengage Learning. All Rights Reserved. May not be scanned, copied or duplicated, or posted to a public
23、ly accessible website, in whole or in part. Example 3.1: Determinants of college GPAInterpretationHolding ACT fixed, another point on high school grade point average is associated with another .453 points college grade point averageOr: If we compare two students with the same ACT, but the hsGPA of s
24、tudent A is one point higher, we predict student A to have a colGPA that is .453 higher than that of student BHolding high school grade point average fixed, another 10 points on ACT are associated with less than one point on college GPAGrade point average at college High school grade point average A
25、chievement test scoreCHAPTER 3 Multiple RegressionAnalysis: Estimation3.2.2 Interpreting the OLS Regression Equation (2/3) S ctionChapt r End 2013 Cengage Learning. All Rights Reserved. May not be scanned, copied or duplicated, or posted to a publicly accessible website, in whole or in part. Example
26、 3.2: Hourly Wage Equationwage1.wf1ls log(wage) c educ exper tenureCHAPTER 3 Multiple RegressionAnalysis: Estimationlog( ) 0.284 0.092 0.0041 0.022Often, 1, and 0.log( ) 0.0041 0.022 0.0261wage educ exper tenureexper tenure educwage exper tenure 3.2.2 Interpreting the OLS Regression Equation (3/3) S
27、 ctionChapt r End 2013 Cengage Learning. All Rights Reserved. May not be scanned, copied or duplicated, or posted to a publicly accessible website, in whole or in part. CHAPTER 3 Multiple RegressionAnalysis: Estimation3.2.3 OLS Fitted Values and ResidualsProperties of OLS on any sample of dataFitted
28、 values and residualsAlgebraic properties of OLS regressionFitted or predicted values Residuals Deviations from regression line sum up to zero Correlations between deviations and regressors are zero Sample averages of y and of the regressors lie on regression line 0u =0i iy u S ctionChapt r Endy y 2
29、013 Cengage Learning. All Rights Reserved. May not be scanned, copied or duplicated, or posted to a publicly accessible website, in whole or in part. One can show that the estimated coefficient of an explanatory variable in a multiple regression can be obtained in two steps:1) Regress the explanator
30、y variable on all other explanatory variables2) Regress on the residuals from this regressionwage1.wf1ls log(wage) c educ exper tenurels educ c exper tenureseries r1=residls log(wage) c r1CHAPTER 3 Multiple RegressionAnalysis: Estimation3.2.4 A “Partialling Out” Interpretation of Multiple Regression
31、 (1/3) S ctionChapt r End0.lo 09g( ) 0.284 0.0041 02 .022wage educ exper tenure log( ) 1.623 0. 92 10wage r 2013 Cengage Learning. All Rights Reserved. May not be scanned, copied or duplicated, or posted to a publicly accessible website, in whole or in part. Why does this procedure work?The residual
32、s from the first regression is the part of the explanatory variable that is uncorrelated with the other explanatory variablesls educ c exper tenureseries r1=residThe slope coefficient of the second regression therefore represents the isolated effect of the explanatory variable on the dep. Variablels
33、 log(wage) c r1CHAPTER 3 Multiple RegressionAnalysis: Estimation3.2.4 A “Partialling Out” Interpretation of Multiple Regression (2/3) S ctionChapt r End 2013 Cengage Learning. All Rights Reserved. May not be scanned, copied or duplicated, or posted to a publicly accessible website, in whole or in pa
34、rt. CHAPTER 3 Multiple RegressionAnalysis: Estimation 0 1 2 21 1 2 1 1 121 11 1 21 1 1 1 1 .The slope can be otained as follows. Regressing on ,., gets . Appendix 3A.2 proves this result.i i i k ikk i i ii i ii i iy x x xx x x x x rr y rr r y y r r 3.2.4 A “Partialling Out” Interpretation of Multipl
35、e Regression (3/3)S ctionChapt r End 2013 Cengage Learning. All Rights Reserved. May not be scanned, copied or duplicated, or posted to a publicly accessible website, in whole or in part. CHAPTER 3 Multiple RegressionAnalysis: Estimation3.2.5 Comparison of Simple and Multiple Regression Estimates 0
36、111 1 1 2 1 2 20 12 0 1 11 1 2 1 correct model:omitted a variable:, where . If =0, and/or 0.y x xy xx x 80.12 5.52 0.24383.08 5.865.86 is near 5.52, and 0.243 is relatively large. Why?prate mrate ageprate mrate Example 3.3 Participation in 401(k) Pension Plansmrate = the amount the firm contributes
37、to a workers fund for each dollar the worker;prate = the percentage of eligible workers having a 401(k) account.S ctionChapt r End 2013 Cengage Learning. All Rights Reserved. May not be scanned, copied or duplicated, or posted to a publicly accessible website, in whole or in part. Decomposition of t
38、otal variationR-squaredAlternative expression for R-squared Notice that R-squared can only increase if another explanatoryvariable is added to the regression. This algebraic fact follows because, by definition, the sum of squared residuals never increases when additional regressors are added to the
39、model.R-squared is equal to the squaredcorrelation coefficient between theactual and the predicted value ofthe dependent variableCHAPTER 3 Multiple RegressionAnalysis: Estimation3.2.6 Goodness of Fit (1/3) 2 2 21 1 1 n n ni i ii i iy y y y u 20 1R 22 ,R cor y y S ctionChapt r End 2013 Cengage Learni
40、ng. All Rights Reserved. May not be scanned, copied or duplicated, or posted to a publicly accessible website, in whole or in part. Example: Explaining arrest recordsInterpretation:Proportion prior arrests +0.5 ! -.075 = -7.5 arrests per 100 menMonths in prison +12 ! -.034(12) = -0.408 arrests for g
41、iven manQuarters employed +1 ! -.104 = -10.4 arrests per 100 menNumber of times arrested 1986 Proportion prior arreststhat led to conviction Months in prison 1986 Quarters employed 1986CHAPTER 3 Multiple RegressionAnalysis: Estimation3.2.6 Goodness of Fit (2/3) S ctionChapt r End 2013 Cengage Learni
42、ng. All Rights Reserved. May not be scanned, copied or duplicated, or posted to a publicly accessible website, in whole or in part. Example: Explaining arrest records (cont.)An additional explanatory variable is added:Interpretation:Average prior sentence increases number of arrests (?)Limited addit
43、ional explanatory power as R-squared increases by littleGeneral remark on R-squaredEven if R-squared is small (as in the given example), regression may still provide good estimates of ceteris paribus effectsAverage sentence in prior convictionsR-squared increases only slightlyCHAPTER 3 Multiple Regr
44、essionAnalysis: Estimation3.2.6 Goodness of Fit (3/3) S ctionChapt r End 2013 Cengage Learning. All Rights Reserved. May not be scanned, copied or duplicated, or posted to a publicly accessible website, in whole or in part. CHAPTER 3 Multiple RegressionAnalysis: Estimation3.2.7 Regression through th
45、e Origin The decomposition of the total variation in y usually does not hold. q R2 might be negative. q some economists propose to calculate R2 as the squared correlation coefficient between the actual and fitted values of y. The cost of estimating an intercept when it is truly zero. S ctionChapt r
46、End 2013 Cengage Learning. All Rights Reserved. May not be scanned, copied or duplicated, or posted to a publicly accessible website, in whole or in part. CHAPTER 3 Multiple RegressionAnalysis: Estimation3.3 The Expected Value of the OLS Estimators3.3.2 Including Irrelevant Variables3.3.3 Omitted Va
47、riable Bias3.3.1 Assumptions and Unbiasedness of OLS Chapter End 2013 Cengage Learning. All Rights Reserved. May not be scanned, copied or duplicated, or posted to a publicly accessible website, in whole or in part. Standard assumptions for the multiple regression modelAssumption MLR.1 (Linear in pa
48、rameters)Assumption MLR.2 (Random sampling) In the population, the relation-ship between y and the expla-natory variables is linear, so is between y and disturbance.The data is a random sample drawn from the population Each data point therefore follows the population equation CHAPTER 3 Multiple Regr
49、essionAnalysis: Estimation3.3.1 Assumptions and Unbiasedness of OLS (1/6) 0 1 1 2 2 . k ky x x x u SectionChapter End 2013 Cengage Learning. All Rights Reserved. May not be scanned, copied or duplicated, or posted to a publicly accessible website, in whole or in part. Standard assumptions for the mu
50、ltiple regression model (cont.)Assumption MLR.3 (No perfect collinearity)Remarks on MLR.3The assumption only rules out perfect collinearity/correlation bet-ween explanatory variables; imperfect correlation is allowedIf an explanatory variable is a perfect linear combination of other explanatory vari
51、ables it is superfluous and may be eliminatedConstant variables are also ruled out (collinear with intercept)nk+1In the sample (and therefore in the population), noneof the independent variables is constant and there areno exact relationships among the independent variables“CHAPTER 3 Multiple Regres
52、sionAnalysis: Estimation3.3.1 Assumptions and Unbiasedness of OLS (2/6) SectionChapter End 2013 Cengage Learning. All Rights Reserved. May not be scanned, copied or duplicated, or posted to a publicly accessible website, in whole or in part. Example for perfect collinearity: small sampleExample for
53、perfect collinearity: relationships between regressorsIn a small sample, avginc may accidentally be an exact multiple of expend; it will notbe possible to disentangle their separate effects because there is exact covariationEither shareA or shareB will have to be dropped from the regression because
54、thereis an exact linear relationship between them: shareA + shareB = 1CHAPTER 3 Multiple RegressionAnalysis: Estimation3.3.1 Assumptions and Unbiasedness of OLS (3/6) SectionChapter End 2013 Cengage Learning. All Rights Reserved. May not be scanned, copied or duplicated, or posted to a publicly acce
55、ssible website, in whole or in part. Standard assumptions for the multiple regression model (cont.)Assumption MLR.4 (Zero conditional mean)In a multiple regression model, the zero conditional mean assumption is much more likely to hold because fewer things end up in the errorExample: Average test sc
56、ores The value of the explanatory variables must contain no information about the mean of the unobserved factorsIf avginc was not included in the regression, it would end up in the error term; it would then be hard to defend that expend is uncorrelated with the error CHAPTER 3 Multiple RegressionAna
57、lysis: Estimation3.3.1 Assumptions and Unbiasedness of OLS (4/6) SectionChapter End 2013 Cengage Learning. All Rights Reserved. May not be scanned, copied or duplicated, or posted to a publicly accessible website, in whole or in part. Discussion of the zero mean conditional assumptioncov(u, xj)=0, j
58、=1, , kFunctional form misspecification, omitted variables, measurement error, and simultaneous equations can cause cov(u, xj)0.Explanatory variables that are correlated with the error term are called endogenous; endogeneity is a violation of assumption MLR.4Explanatory variables that are uncorrelat
59、ed with the error term are called exogenous; MLR.4 holds if all explanat. var. are exogenousExogeneity is the key assumption for a causal interpretation of the regression, and for unbiasedness of the OLS estimatorsCHAPTER 3 Multiple RegressionAnalysis: Estimation3.3.1 Assumptions and Unbiasedness of
60、 OLS (5/6) SectionChapter End 2013 Cengage Learning. All Rights Reserved. May not be scanned, copied or duplicated, or posted to a publicly accessible website, in whole or in part. Theorem 3.1 (Unbiasedness of OLS)Unbiasedness is an average property in repeated samples; in a given sample, the estima
61、tes may still be far away from the true valuesPROOF:CHAPTER 3 Multiple RegressionAnalysis: Estimation 21 1 1 2 1 1 1 11 11 1 1 #i i ii i ir y rr u rEE E E XX X 3.3.1 Assumptions and Unbiasedness of OLS (6/6) SectionChapter End 2013 Cengage Learning. All Rights Reserved. May not be scanned, copied or
62、 duplicated, or posted to a publicly accessible website, in whole or in part. Including irrelevant variables in a regression model= 0 in the population No problem because .However, including irrevelant variables may increase sampling variance.CHAPTER 3 Multiple RegressionAnalysis: Estimation3.3.2 In
63、cluding Irrelevant Variables SectionChapter End 2013 Cengage Learning. All Rights Reserved. May not be scanned, copied or duplicated, or posted to a publicly accessible website, in whole or in part. Omitting relevant variables: the simple caseOmitted variable biasConclusion: All estimated coefficien
64、ts will be biased If x1 and x2 are correlated, assume a linear regression relationship between themIf y is only regressed on x 1 this will be the estimated intercept If y is only regressed on x1, this will be the estimated slope on x1 error term CHAPTER 3 Multiple RegressionAnalysis: Estimation3.3.3
65、 Omitted Variable Bias (1/6)True model (contains x1 and x2)Estimated model (x 2 is omitted) SectionChapter End 2013 Cengage Learning. All Rights Reserved. May not be scanned, copied or duplicated, or posted to a publicly accessible website, in whole or in part. Example: Omitting ability in a wage eq
66、uationWhen is there no omitted variable bias?If the omitted variable is irrelevant or uncorrelated Will both be positive The return to education will be overestimated because . It will look as if people with many years of education earn very high wages, but this is partly due to the fact that people with more education are also more able on average.CHAPTER 3 Multiple RegressionAnalysis: Estimation3.3.3 Omitted Variable Bias (2/6) SectionChapter End 2013 Cengage Learning. All Rights Reserved. May
- 溫馨提示:
1: 本站所有資源如無(wú)特殊說(shuō)明,都需要本地電腦安裝OFFICE2007和PDF閱讀器。圖紙軟件為CAD,CAXA,PROE,UG,SolidWorks等.壓縮文件請(qǐng)下載最新的WinRAR軟件解壓。
2: 本站的文檔不包含任何第三方提供的附件圖紙等,如果需要附件,請(qǐng)聯(lián)系上傳者。文件的所有權(quán)益歸上傳用戶所有。
3.本站RAR壓縮包中若帶圖紙,網(wǎng)頁(yè)內(nèi)容里面會(huì)有圖紙預(yù)覽,若沒(méi)有圖紙預(yù)覽就沒(méi)有圖紙。
4. 未經(jīng)權(quán)益所有人同意不得將文件中的內(nèi)容挪作商業(yè)或盈利用途。
5. 裝配圖網(wǎng)僅提供信息存儲(chǔ)空間,僅對(duì)用戶上傳內(nèi)容的表現(xiàn)方式做保護(hù)處理,對(duì)用戶上傳分享的文檔內(nèi)容本身不做任何修改或編輯,并不能對(duì)任何下載內(nèi)容負(fù)責(zé)。
6. 下載文件中如有侵權(quán)或不適當(dāng)內(nèi)容,請(qǐng)與我們聯(lián)系,我們立即糾正。
7. 本站不保證下載資源的準(zhǔn)確性、安全性和完整性, 同時(shí)也不承擔(dān)用戶因使用這些下載資源對(duì)自己和他人造成任何形式的傷害或損失。
相關(guān)資源
更多